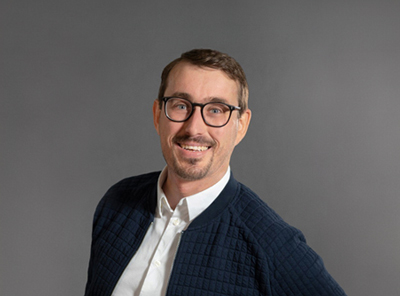
Mechanistic modeling is considered a part of smart process development, which is a collection of approaches to get better process outcomes and speed up process development.
This week, Bioprocess Development Forum spoke with Tobias Hahn, PhD, co-founder and CEO of GoSilico, about the opportunities of mechanistic modeling in bioprocess development, now and in the future.
Can you briefly describe what mechanistic modeling really is, and what the main benefits are when using it in bioprocess development?
Mechanistic models are based on the fundamental laws of natural sciences. Physical and biochemical principles constitute the model equations. Only few experimental data are needed to calibrate the model to the particular process. Since natural laws are generally valid, mechanistic models can extrapolate far beyond the calibration space. After calibration, process parameters and the actual process set-up can easily be changed in silico without further experimentation. This opens a wide range of model applications—from early-stage process development via process characterization to process monitoring and control.
GoSilico has developed the ChromX™ software. What are the main advantages of using the software?
The nature of mechanistic models requires some amount of expert knowledge. ChromX is suited to facilitate this modeling approach as far as possible by hiding the underlying mathematical equations behind the graphical user interface and by using preset options in the mathematical equation solver. Also, ChromX offers multiple helpful features, including the ability to facilitate data import from ÄKTA™ systems and finding correlations between process parameters and quality attributes. But the inner workings of ChromX are not as impressive compared with what our users have achieved with the software.
Indeed, a variety of case studies have been presented at conferences that would have been impossible with a purely experimental approach. I am thinking, for example, of explaining significant changes in the chromatograms of scale down models, or of converting batch processes to continuous multi-column processes entirely in silico. In many companies, ChromX has also enabled a new quality of knowledge management throughout the entire product life cycle, where models are handed over from early process development to late stage and production instead of ring binders.
But especially in the current COVID-19 situation, the benefits of mechanistic modeling become increasingly obvious. Wet lab work, for example, is difficult to carry out in times of social distancing and home office.
How do you believe mechanistic modeling in process development will develop in the future?
Mechanistic models are already considered being a game changer for the industry and their applications will be widespread in the coming years. As the time-to-market pressure increases, companies are forced to continuously speed up their process development. One of the most important applications that I anticipate is the early sort out of hard to purify molecules. As mechanistic modeling uses only few calibration experiments, the realization that uneconomic purification process can be found early. This might have an even bigger economic impact than quickly identifying optimal process conditions for an easy separation.
I also foresee that we will see the appearance of predictive mechanistic models for further unit operations very soon as well as the integration with other modeling disciplines. Molecular dynamics simulations increase our scientific understanding, while a hybrid of mechanistic and statistic models allows to better cope with uncertainty, noise and trends over production cycles. Once the models have been calibrated and validated, they can be used as so-called digital twinsto monitor and control production, and support troubleshooting.
What do you think, how will in silico solutions influence regulatory requirements such as risk management or process characterization under the QbD-paradigm?
We are already observing a change that the regulatory authorities increasingly demand for process understanding. This is not coming as a surprise. A systematic way of relating material attributes and process parameters to drug product quality attributes should be self-understood. Purely data-driven approaches then have a hard time explaining why those relationships should be proportional, linear or quadratic. As mechanistic models are based on scientific principles, they can naturally prove the required relationships and resulting in process understanding. The authorities seem to be open towards this approach.
Another argument is that simulations can predict thousands of different process scenarios within minutes. That allows for more thoroughness during process characterization for example. As process characterization and validation is not done at production scale but typically with bench-top systems, arguments must be found why a bench-top model should be representative for the production scale. Mechanistic models can also help here. Only the fluid dynamic parameters must be adapted to the new scale. The actual interactions of molecules with the ligands on the resin do not change.
In summary, I am convinced that soon, modeling will be an asset in de-risking drug manufacturing but also the process of filing a new drug application.
Observing recent publications and trends, many voices within the biopharmaceutical industry seem to be convinced that bioprocessing 4.0 and the concept of digital twins will assert themselves. What could accelerate this process?
In almost all industries, the overall level of digitalization is increasing—with our industry being a bit more conservative than others. The data flows that we envision for efficient model building are not yet in place. Column and resin properties as well as buffer recipes are often not easily accessible.
Even assigning fraction analyses to the right time point in the chromatogram often needs extra work. Theses fraction analyses are currently needed to reveal the individual peak shapes of complex multi-component separations. The implementation of advanced process analytical technologies (PAT), especially online real-time monitoring could further accelerate the roll-out of mechanistic modeling in the biopharmaceutical industry.
Once the first molecules, whose development process was mainly driven by modeling, have passed regulatory approval, I expect a strong demand for the type of modelling that we are doing. Based on our experience, many companies in the industry are already preparing themselves and employ dedicated modeling experts – and there are more to come. And I’m not saying that as a maths graduate. The next generation of process developers will be digital natives who consider simulations essential to their work.